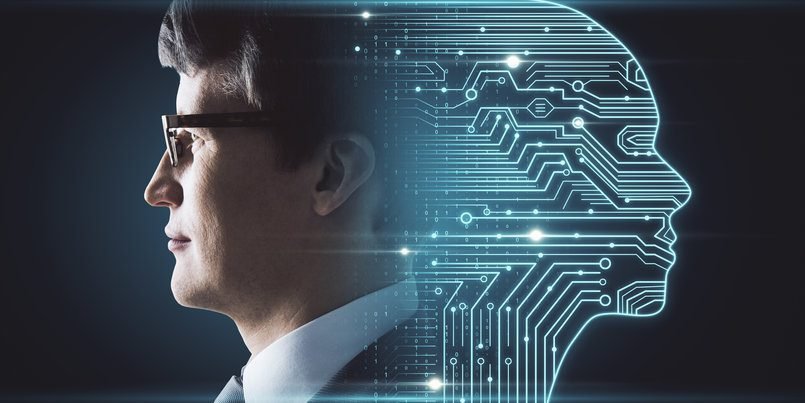
Real life tips and learnings of applying artificial intelligence in marketing
This post is by Anton Buchner, a senior consultant with TrinityP3. Anton is one of Australia’s leaders in data-driven marketing. Helping navigate through the bells, whistles and hype to identify genuine marketing value when it comes to technology, digital activity, and the resulting data footprint.
Trends abound in Marketing. It’s an exciting part of the discipline’s continual evolution.
And the Artificial Intelligence (AI) space is no exception.
Over the past few years we’ve seen the rise and rise of AI discussion and solutions in marketing. From identifying new market opportunities through machine learning, and driving demand with intelligent research and targeting, through to assistants, image recognition, and personalised product recommendations.
I have spent the past month talking to a wide variety of industry thought leaders and experts in the AI space – from business, agency, and tech vendor perspectives. With the aim of identifying how Australian marketers are using AI solutions to enhance and anticipate consumer interaction. In this post, I would like to share some of their experiences and learnings to date.
However, before we jump in, as I’m sure most of you know, AI dates back decades. Let’s take a quick look back at how AI emerged.
Snapshot history
During WW2, British computer scientist Alan Turing cracked the German armed forces’ ‘Enigma’ code with an ‘intelligent’ machine. He had built on the work of Konrad Zuse, Warren McCullough, Walter Pitts and many others around the first working program-controlled computers, game theory, and the logical behaviour of nervous activity and connected neural networks.
In 1950 Turing released his landmark “Computing Machinery and Intelligence” paper, outlining the Turing Test. A test of a machine’s ability to exhibit ‘intelligent’ behaviour. In short, if a machine could carry on a conversation (over a teleprinter) that was indistinguishable from a conversation with a human being, then it was reasonable to say that the machine was “thinking”.
In 1956 the term ‘Artificial Intelligence’ was coined by a handful of mathematicians and scientists in a brainstorming workshop at Dartmouth College, New Hampshire. Called “The Dartmouth Summer Research Project on Artificial Intelligence”, the workshop was widely considered to be the founding event of AI as a field. Although no specific theory or methodology was agreed, the researchers continued with a 2 month study of AI with the shared vision that computers can be made to perform intelligent tasks.
During the ‘space race’ to achieve firsts in spaceflight capability, the long-term Stanford Cart project took many forms from around 1960 to 1980. It was originally designed to test what it would be like to control a lunar rover from Earth and was eventually reconfigured by Hans Moravec as the first computer-controlled, autonomous vehicle. Successfully traversing a chair-filled room and circumnavigating the Stanford AI Lab.
Human fascination from a philosophical sense was fast becoming a reality, and in the 1970s, Japan started to lead the way with advancements in machine learning algorithms in robotic systems.
However, it was IBM in 1997 who shocked the world with its Deep Blue chess-playing computer system (originally called ChipTest, then Deep Thought) that defeated the reigning world chess champion at the time, Garry Kasparov.
After major surges, AI funding started to dry up after the dotcom burst in the early 2000s.
However, as the digital landscape became mainstream, and computing power advanced again, AI has now become the next mega-trend – after ‘mobile first’.
Now we see regular headlines around the world from Alibaba, Apple, Google, Amazon, Tesla, Uber, Microsoft, Salesforce, Facebook and many other businesses, about advancements in autonomous mobility, affective computing, artificial co-workers, chatbots, cognitive computing and intelligent personal assistants.
One of the more memorable ones being about Sophia, a social humanoid robot developed by Hong Kong based company Hanson Robotics. Sophia’s face and voice were modelled on the actress Audrey Hepburn, and she was unveiled at South by Southwest (SXSW) in 2016. A year later Sophia became the first robot to receive citizenship – granted by Saudi Arabia.
Exploring solutions for marketers
One of the biggest applications of AI in marketing is for more intelligent data analytics, specifically predictive analytics to better understand customers.
In late 2016 Salesforce launched Salesforce Einstein, artificial intelligence embedded in the Salesforce Platform, claiming Salesforce to be the world’s smartest CRM.
Einstein is a layer of artificial intelligence that delivers predictions and recommendations based on people’s interactions with technology. Marketers and sales teams can use Einstein analytics to identify insights to automate responses and actions, and make employees more productive. For example deals can be identified in terms of prediction to close, giving teams the right ones to focus on.
Einstein is also being used in social media. For example, when a customer redeems an offer by taking a picture with the product and posting it to social media, Einstein uses image recognition to identify the company product and auto-responds to the customer. Einstein can also understand the intent behind the user’s social post and respond with offers for a particular brand.
At the same time, Adobe responded with its Adobe Sensei product – AI and machine learning for customer experiences. Also particularly useful within the Adobe Creative Cloud & Campaign ecosystems where it can used to identify better imagery to drive conversions, allow you to write an email and have it translated into multilingual options, and modifying length of copy for different channels.
In 2018 Alibaba launched its own AI copywriting tool that can write 20,000 lines of copy and thousands of ads in a second. Launched through its digital marketing technology and big data unit, Alimama. Esprit was one of the first brands to test it in Taobao and Tmall, adjusting the length and tone of its advertising copy and choosing whether they wanted the ads to be “promotional, functional, fun, poetic or heart-warming.”
One of the challenges in all of the above is not just understanding human behaviour, but also understanding and interpreting human language.
So as we move from hype to reality, here’s what some business leaders are doing to test the waters.
Dave King and his new company Move37 make a move into augmented creativity
Dave King, cofounder of Move37, touched on some fascinating points in terms of using AI in partnership with people in the process of problem solving and creativity.
Firstly, I asked Dave about the meaning behind Move37, and then what they’re aiming to do in the AI space.
For those of you who may not know, Dave cofounded The Royals agency along with Andrew Siwka, Stephen O’Farrell and Nick Cummins back in 2010. And he remains a Director and advises them on innovation, AI and other emerging technologies.
He says his love for all things digital stemmed from the first text browser that he used with dial-up when dating a girl at Monash University in the early 90’s. She was doing a long distance education course, and Dave was starting to tinker with accessing servers across the world on this thing called the internet. He ended up building the first website for the Arts Faculty as a Psychology Course Advisor under direction from the Dean.
Dave continued in his career to create websites for an ISP, then went to “an awesome job” reviewing video games at Hyper Magazine (Next Media), worked at MSN in the late ‘90s followed by exploring emerging technology including mobile TV and IPTV with Sensis.
Always fascinated by human behaviour, Dave started looking at how machines could work in different ways in the creative world.
The name, Move37, was inspired by a move in an historic match in South Korea in 2016 of the 2,500 year-old game Go. Between 18-time world champion and Korean Grandmaster, Lee Sedol, and Google’s artificially intelligent Go-playing computer system, AlphaGo – designed by a team of researchers at DeepMind, a London AI lab now owned by Google.
AlphaGo’s 37th move in the match’s second game was first described as “very unusual” and thought to be a mistake, but then realised it was simply “beautiful” and “creatively genius” in the way that it turned the game. It had calculated that there was a tiny one-in-ten-thousand chance of a human ever playing the move, and played it anyway. AlphaGo then went on to win the game and the overall match by four games to one.
Dave talked about one of the exciting opportunities for AI. How to inject some novelty and randomness to help people think laterally. Allowing creative ideation to be more than just by humans. Thought of as more in partnership with machines. And says he’s “testing using machine learning, data mining and natural language processing to augment ideation, conceptual creativity and invention”.
Move 37 is working with a number of algorithms, models and datasets including GPT-2, developed by Open AI. GPT-2 is state of the art in the field of text generation, but Move 37’s ambitions are to bring common sense reasoning and causality to its AI.
Open AI is a non-profit AI research company comprised of a team of hundred people based in San Francisco with the mission to ensure that artificial general intelligence (AGI) benefits all humanity. The GPT-2 model trained itself on 8 million webpages including unstructured conversations on topics in Reddit threads, and the related interesting external links that people have recommended that have received at least 3 upvotes.
But Dave sees AI in its very early days in the creative realm. His focus is on augmenting machines and knowledge workers. People whose role requires thinking for a living – utilising their experience and the ability to collect and analyse data for decision making and action (ie: marketers, architects, urban planners, researchers, scientists, lawyers, academics, engineers etc).
The main focus of Dave’s work at Move37 is creating an augmented creativity engine. A platform or capability to brainstorm in new and interesting ways, which can make sense of the mass of information available, and distill it in creative ways.
Dave and his team’s approach centres around creative thinking and critical thinking, with creative reasoning capability to improve people’s ides by introducing new lenses, new perspectives, new directions.
He sees humans as currently being the curators of what might work when AI tools offer solutions.
Whilst machines and AI are relatively good at correlations between things, AI hasn’t been great at explaining where recommendations come from, or the transparency behind causality. And therefore one of the major issues is the lack of trust in AI recommendations in the realm of major problem solving.
However, Dave sees this as a big opportunity. To be focused more on a common sense understanding of the world and the data. Looking at developing tools that understand causality, frame relationships, and understand patterns and analogy.
So for knowledge workers, it’s about artificial creativity augmenting their expertise.
Dave is reverse engineering creative process and taking the popular practices in commercial creativity where there’s a problem that people need to work through to create a solution.
He sees creativity as putting things together in novel ways, and believes creativity can be learned. It can be developed, practiced, iterated and improved. So the platform that he’s working on will help people get better at problem solving by understanding the relationships and connections between things. Users will have the chance to combine concepts and knowledge in novel ways to identify relationships that are interesting and that weren’t readily identifiable or thought of before.
Mauricio Perez outlines how to get your chatbot right
Mauricio Perez is a Human Centred Design (HCD) strategist, specialising in Service Design, User Experience (UX) and Customer experience (CX).
Mauricio helped assist nib health funds (nib) to become the first Australian health insurer to introduce AI technology (a chatbot called nibby utilising Amazon Alexa) to assist health insurance enquiries.
The chatbot provides customers with access to simple responses regarding their health insurance. And unlike many other chatbots, nibby is integrated into nib’s web platform, allowing it to intelligently move customers to the right sales or claims consultant as a customer’s query becomes more complex, and to offer assistance during key customer service moments.
Mauricio didn’t think it would be difficult to design a conversational interface flow to get people to the right place. What he learned, however, was quite the opposite. Mapping the flows with text seemed easy enough until he realised the enormous range of language constructs that people used in even responding to a bot greeting in the context of getting what they needed.
For example, in concept testing, some people assumed they were talking to a real human and responded in terms that were not understood by a nascent nibby. The variants in natural language varied by English capability, education levels, jargonistic terms, emotional states, device usage and so on. At times, it wasn’t explicit that the human was conversing with a bot and when they found out, some experienced a sense of betrayal and distrust. For best practice, always ensure that the bot presents itself as a non-human and announce its limitations so that people have a realistic expectation.
Something else that tended to annoy people was the lack of variety in the type of responses when there was an unknown input. When people expressed a need and the bot did not recognise the words, it gave a limited number of responses which tended to affect the level of trust people had when they kept getting the same response. Instead, try to guide the user in responses that will set them up for success.
Another challenge was that the technology had already been decided upon. This meant that the flexibility of what could be delivered was already being compromised. In hindsight, it would have been a better exercise to perform a “Wizard of Oz” test. This is where somebody emulates a bot in another room and captures the common ways in which an unknowing human would interface with them. Only then once the conversation variables are captured, should a technology decision be made knowing the customers’ conversational contexts and the parameters that the technology should flex towards.
In addition, this test would determine the kind of personality would best suit a future chatbot and even why it might vary its language in some contexts. Should the conversation be playful or dry? Understated or enthusiastic? Formal or Informal?
Also, he learned that conversation decisions should be made collaboratively. Copywriters should be working together with customer support staff and developers. A good collaborative tool like LucidChart or BotMock should be used.
Mauricio highlighted that “integrating with backend systems and humans became one of the biggest challenges. The logic of the front end conversation had to match the logic at the back end of the system. Ensuring that the customer experience was being enhanced and not breaking down.
Thinking about the service of delivering a chatbot, there were some important lessons learned to help guide the launch of a chatbot, especially when preparing for the human behaviours and technology chosen. There was another lesson learned when the chatbot was launched: Human Hand-overs. This is when the bot needs to hand the conversation over to a human to continue or when things go wrong.
- Ensure your bot captures all the different ways somebody might ask for a real human.
- Ensure that the systems being used to hand-over are quick, reliable and easy to use from a contact centre perspective. Otherwise, people will disengage from the conversation by the time a real human reads the conversation and gets back to the user.
Finally, it was tempting to start adding UI (user interface) elements like buttons or form components into the conversation interface. While this made it easier to get the user to get them to their destination, this made the chat interface less accessible and limited the capability of NLP (Natural Language Processing). The addition of UI into the conversation is then further limited when the logic needs to be scaled or adapted to a voice interface in the future.
Read more about the Nibby Case study, and Chatbot and AI Design principles